Lecture 6, Heat transports#
Two-dimensional advection-diffusion of heat
import xarray as xr
import numpy as np
import matplotlib.pyplot as plt
from ipywidgets import interact, interactive, fixed, interact_manual
import ipywidgets as widgets
from IPython.display import HTML
from IPython.display import display
1) Background: two-dimensional advection-diffusion#
1.1) The two-dimensional advection-diffusion equation#
Recall from the last Lecture that the one-dimensional advection-diffusion equation is written as
where
The two-dimensional advection diffusion equation simply adds advection and diffusion operators acting in a second dimensions
where
Throughout the rest of the Climate Modelling module, we will consider
1.2) Reminder: Multivariable shorthand notation#
Conventionally, the two-dimensional advection-diffusion equation is written more succintly as
using the following shorthand notation.
The gradient operator is defined as
such that
The Laplacian operator
such that
The divergence operator is defined as
Note: Since seawater is largely incompressible, we can approximate ocean currents as a non-divergent flow, with
\begin{align} \vec{u} \cdot \nabla T&= u\frac{\partial T(x,y,t)}{\partial x} + v\frac{\partial T(x,y,t)}{\partial y}\newline &= u\frac{\partial T}{\partial x} + v\frac{\partial T}{\partial y} + T\left(\frac{\partial u}{\partial x} + \frac{\partial v}{\partial y}\right)\newline &= \left( u\frac{\partial T}{\partial x} + T\frac{\partial u}{\partial x} \right) + \left( v\frac{\partial T}{\partial y} + \frac{\partial v}{\partial y} \right) \newline &= \frac{\partial (uT)}{\partial x} + \frac{\partial (vT)}{\partial y}\newline &= \nabla \cdot (\vec{u}T) \end{align}
using the product rule (separately in both
This lets us finally re-write the two-dimensional advection-diffusion equation as:
which is the form we will use in our numerical algorithm below.
2) Numerical implementation#
2.1) Discretizing advection in two dimensions#
In the last lecture we saw that in one dimension we can discretize a first-partial derivative in space using the centered finite difference
In two dimensions, we discretize the partial derivative the exact same way, except that we also need to keep track of the cell index
The x-gradient kernel below, is shown below, and is reminiscent of the edge-detection or sharpening kernels used in image processing and machine learning:
plt.imshow([[-1,0,1]], cmap=plt.cm.RdBu_r)
plt.xticks([0,1,2], labels=[-1,0,1]);
plt.yticks([]);
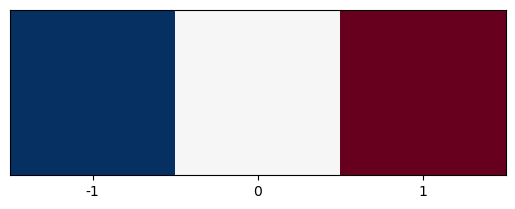
The first-order partial derivative in
Its kernel is shown below.
plt.imshow([[-1,-1],[0,0],[1,1]], cmap=plt.cm.RdBu);
plt.xticks([]);
plt.yticks([0,1,2], labels=[1,0,-1]);
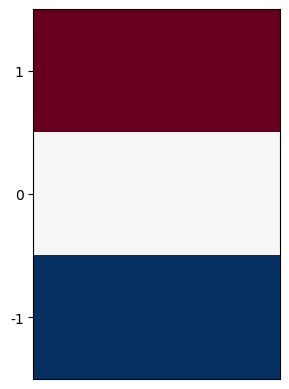
Now that we have discretized the two derivate terms, we can write out the advective tendency for computing
We implement this as a the advect
function. This computes the advective tendency for the
xkernel = np.zeros((1,3))
xkernel[0, :] = np.array([-1,0,1])
ykernel = np.zeros((3,1))
ykernel[:, 0] = np.array([-1,0,1])
class OceanModel:
def __init_(self,T, u, v, deltax, deltay):
self.T = [T]
self.u = u
self.v = v
self.deltax = deltax
self.deltay = deltay
self.j = np.arange(0, self.T[0].shape[0])
self.i = np.arange(0, self.T[0].shape[1])
def advect(self, T):
T_advect = np.zeros((self.j.size, self.i.size))
for j in range(1, T_advect.shape[0] - 1):
for i in range(1, T_advect.shape[1] - 1):
T_advect[j, i] = -(self.u[j, i] * np.sum(xkernel[0, :] * T[j, i-1 : i + 2])/(2*self.deltax) +
self.v[j, i] * np.sum(ykernel[:, 0] * T[j-1:j+2, i])/(2*self.deltay))
return T_advect
2.2) Discretizing diffusion in two dimensions#
Just as with advection, the process for discretizing the diffusion operators effectively consists of repeating the one-dimensional process for
The corresponding
plt.imshow([[1,1],[0,0],[1,1]], cmap=plt.cm.RdBu_r);
plt.xticks([]);
plt.yticks([0,1,2], labels=[1,-1,1]);
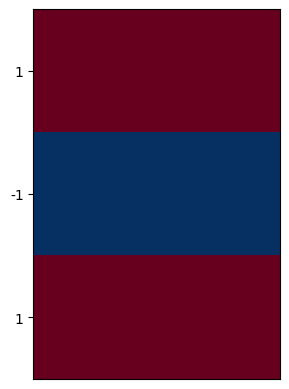
plt.imshow([[1,0,1]], cmap=plt.cm.RdBu_r);
plt.xticks([0,1,2], labels=[1,-1,1]);
plt.yticks([]);
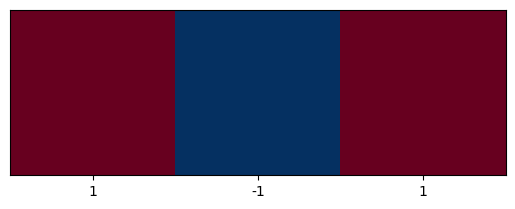
xkernel_diff = np.zeros((1, 3))
xkernel_diff[0, :] = np.array([1,-2,1])
ykernel_diff = np.zeros((3,1))
ykernel_diff[:, 0] = np.array([1,-2,1])
Just as we did with advection, we implement a diffuse function using multiple dispatch:
class OceanModel():
def __init__(self, T, deltat, windfield, grid, kappa):
self.T = [T]
self.u = windfield.u
self.v = windfield.v
self.deltay = grid.deltay
self.deltax = grid.deltax
self.deltat = deltat
self.kappa = kappa
self.i = np.arange(0, self.T[0].shape[1])
self.j = np.arange(0, self.T[0].shape[0])
self.t = [0]
self.diffuse_out = []
self.advect_out = []
self.t_ = []
def advect(self, T):
T_advect = np.zeros((self.j.size, self.i.size))
for j in range(1, T_advect.shape[0] - 1):
for i in range(1, T_advect.shape[1] - 1):
T_advect[j, i] = -(self.u[j, i] * np.sum(xkernel[0, :] * T[j, i-1:i+2])/(2*self.deltax) + \
self.v[j, i] * np.sum(ykernel[:, 0] * T[j-1:j+2, i])/(2*self.deltay))
return T_advect
def diffuse(self, T):
T_diffuse= np.zeros((self.j.size, self.i.size))
for j in range(1, T_diffuse.shape[0] - 1):
for i in range(1, T_diffuse.shape[1] - 1):
T_diffuse[j, i] = self.kappa*(np.sum(xkernel_diff[0, :] * T[j, i-1:i+2])/(self.deltax**2) + \
np.sum(ykernel_diff[:, 0] * T[j-1:j+2, i])/(self.deltay**2))
return T_diffuse
2.3) No-flux boundary conditions#
We want to impose the no-flux boundary conditions, which states that
To impose this, we treat
Thus, we can implement the boundary conditions by updating the temperature of the ghost cells to match their interior-point neighbors:
def update_ghostcells(var):
var[0, :] = var[1, :];
var[-1, :] = var[-2, :]
var[:, 0] = var[:, 1]
var[:, -1] = var[:, -2]
return var
B = np.random.rand(6,6)
plt.pcolor(B)
plt.colorbar()
<matplotlib.colorbar.Colorbar at 0x13a4a7d90>
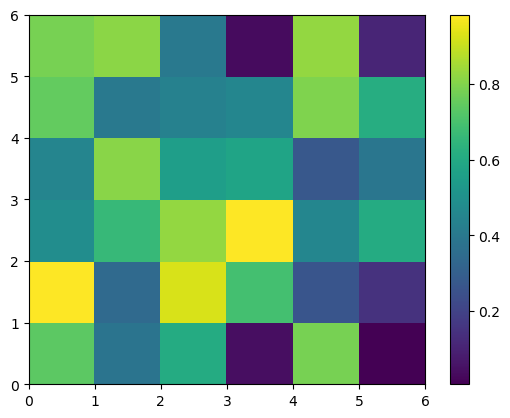
B = update_ghostcells(B)
plt.imshow(B)
plt.colorbar()
<matplotlib.colorbar.Colorbar at 0x13a537640>
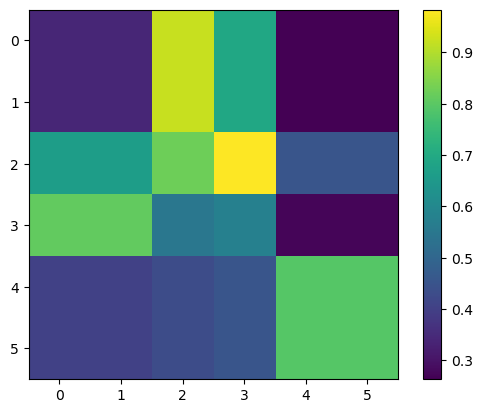
2.4) Timestepping#
class OceanModel():
def __init__(self, T, deltat, windfield, grid, kappa):
self.grid = grid
self.T = [T]
self.u = windfield['u']
self.v = windfield['v']
self.deltat = deltat
self.kappa = kappa
self.omega = 7.2921e-5 # Earth's angular velocity in rad/s
self.f = 2 * self.omega * np.sin(np.deg2rad(grid.lat_grid)) # Coriolis parameter f
self.i = np.arange(0, T.shape[1])
self.j = np.arange(0, T.shape[0])
self.t = [0]
self.diffuse_out = []
self.advect_out = []
self.t_ = []
def update_ghostcells(self, var):
var[0, :] = var[1, :];
var[-1, :] = var[-2, :]
var[:, 0] = var[:, 1]
var[:, -1] = var[:, -2]
return var
def advect(self, T):
T_advect = np.zeros((self.j.size, self.i.size))
for j in range(1, T_advect.shape[0] - 1):
for i in range(1, T_advect.shape[1] - 1):
T_advect[j, i] = -(self.u[j, i] * np.sum(xkernel[0, :] * T[j, i-1:i+2])/(2*self.grid.delta_lon[j,i]) + \
self.v[j, i] * np.sum(ykernel[:, 0] * T[j-1:j+2, i])/(2*self.grid.delta_lat))
return T_advect
def diffuse(self, T):
T_diffuse= np.zeros((self.j.size, self.i.size))
for j in range(1, T_diffuse.shape[0] - 1):
for i in range(1, T_diffuse.shape[1] - 1):
T_diffuse[j, i] = self.kappa*(np.sum(xkernel_diff[0, :] * T[j, i-1:i+2])/(self.grid.delta_lon[j,i]**2) + \
np.sum(ykernel_diff[:, 0] * T[j-1:j+2, i])/(self.grid.delta_lat**2))
return T_diffuse
def timestep(self):
T_ = self.update_ghostcells(self.T[-1].copy())
# Calculate Coriolis forces
f_u = -self.f * self.v
f_v = self.f * self.u
# Update the velocities with the Coriolis effect
self.u += self.deltat * f_u
self.v += self.deltat * f_v
# Proceed with advection and diffusion as before
tendencies = self.advect(T_) + self.diffuse(T_)
T_ += self.deltat * tendencies
# Store the new temperature field
self.T.append(T_)
self.t.append(self.t[-1] + self.deltat)
# You can instantiate the grid outside of the OceanModel like this:
N_lat = 180 # Number of latitude grid points
N_lon = 360 # Number of longitude grid points
grid = Grid(N_lat, N_lon)
# And then create the OceanModel with the new grid:
windfield = {'u': np.random.rand(N_lat, N_lon), 'v': np.random.rand(N_lat, N_lon)}
def create_wind_field(N_lat, N_lon, max_speed=10):
"""
Create a simple zonal wind field for an aquaplanet.
Parameters:
N_lat -- number of latitude grid points
N_lon -- number of longitude grid points
max_speed -- maximum speed of the wind (m/s)
Returns:
windfield -- a dictionary with 'u' and 'v' components of wind
"""
# Create a latitude array that goes from -90 to 90 degrees
latitudes = np.linspace(-90, 90, N_lat)
# Create the zonal wind profile - simple sinusoidal variation
u_wind_profile = max_speed * np.sin(np.deg2rad(latitudes))
# Create the u component of the wind field - it varies with latitude but is constant with longitude
u_wind = np.tile(u_wind_profile, (N_lon, 1)).T
# The v component (meridional wind) is zero in this simple model
v_wind = np.zeros((N_lat, N_lon))
windfield = {'u': u_wind, 'v': v_wind}
return windfield
# Example usage:
N_lat = 180 # number of latitude points
N_lon = 360 # number of longitude points
windfield = create_wind_field(N_lat, N_lon)
kappa = 1e-6 # Example diffusivity
deltat = 3600 # Example time step in seconds
[[5. 4.99923423 4.99693714 ... 4.99693714 4.99923423 5. ]
[5.52649688 5.52573111 5.52343402 ... 5.52343402 5.52573111 5.52649688]
[6.05283158 6.05206581 6.04976873 ... 6.04976873 6.05206581 6.05283158]
...
[6.05283158 6.05206581 6.04976873 ... 6.04976873 6.05206581 6.05283158]
[5.52649688 5.52573111 5.52343402 ... 5.52343402 5.52573111 5.52649688]
[5. 4.99923423 4.99693714 ... 4.99693714 4.99923423 5. ]]
import numpy as np
import matplotlib.pyplot as plt
import cartopy.crs as ccrs
import cartopy.feature as cfeature
# Create latitude array from -90 to 90 degrees
latitudes = np.linspace(-90, 90, N_lat)
# Create an initial temperature distribution with higher temperatures at the Equator
initial_temperature = np.zeros((N_lat, N_lon))
# Assuming a simplistic temperature gradient
for i, lat in enumerate(latitudes):
initial_temperature[i, :] = max(30 - abs(lat)/90 * 60, -30) # Temperature decreases from equator to poles
# Set up the map projection and the figure
fig = plt.figure(figsize=(10, 5))
ax = fig.add_subplot(1, 1, 1, projection=ccrs.PlateCarree())
# Set up the longitude and latitude arrays for the grid
longitudes = np.linspace(-180, 180, N_lon)
latitudes = np.linspace(-90, 90, N_lat)
# Create a meshgrid for the map projection
lon2d, lat2d = np.meshgrid(longitudes, latitudes)
# Plot the data using pcolormesh
temperature_plot = ax.pcolormesh(lon2d, lat2d, initial_temperature,
transform=ccrs.PlateCarree(),
cmap='coolwarm')
# Add coastlines for context
ax.coastlines()
# Add a color bar
cbar = plt.colorbar(temperature_plot, orientation='horizontal', pad=0.05)
cbar.set_label('Temperature (°C)')
# Set map extent (could be global or specific region)
ax.set_global()
# Add gridlines and features
ax.gridlines(draw_labels=True, dms=True, x_inline=False, y_inline=False)
ax.add_feature(cfeature.BORDERS)
# Show the plot
plt.show()
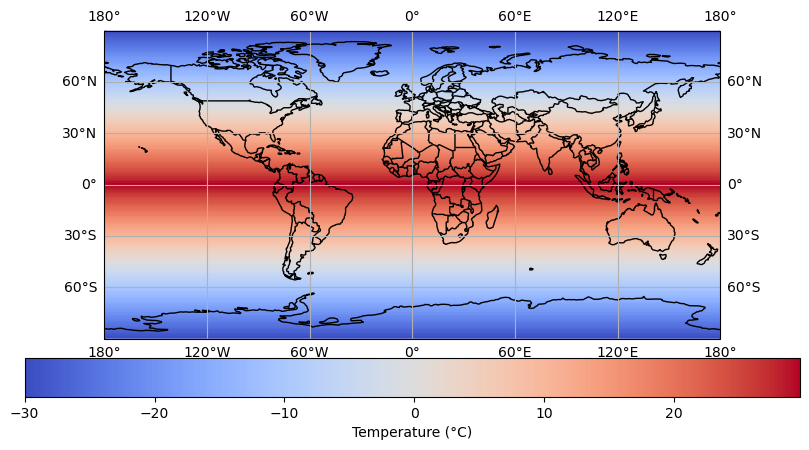
plt.quiver(windfield["u"][::10,::10], windfield["v"][::10,::10])
<matplotlib.quiver.Quiver at 0x172090250>
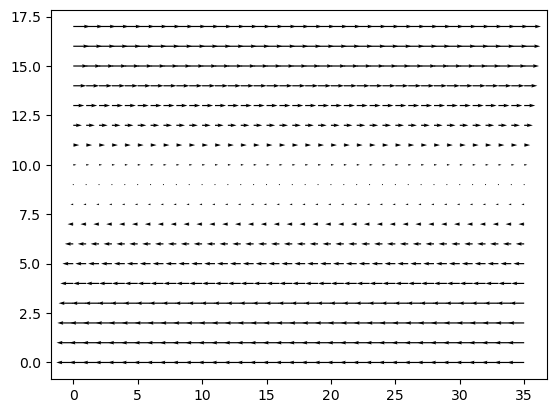
model = OceanModel(initial_temperature, deltat, windfield, grid, kappa)
grid.delta_lon.shape
(180, 360)
model.timestep()
plt.pcolor(model.T[2])
<matplotlib.collections.PolyCollection at 0x28613fa90>
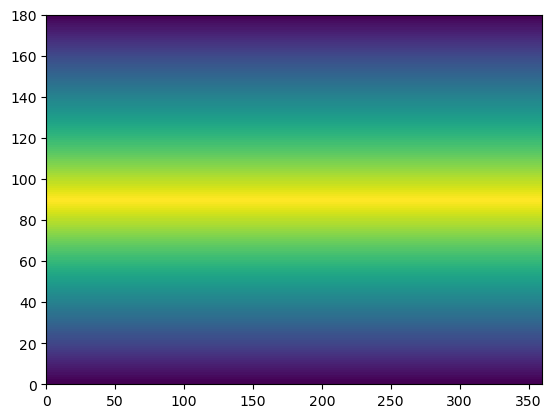
import numpy as np
class Grid:
""" Creates grid for ocean model on a spherical surface (aqua planet). """
def __init__(self, N_lat, N_lon, radius=6371e3):
"""
Args:
N_lat: Number of latitude grid points
N_lon: Number of longitude grid points
radius: Radius of the planet (default is Earth's radius in meters)
"""
self.radius = radius
# Latitude ranges from -90 to 90 degrees
self.lat = np.linspace(-90, 90, N_lat)
# Longitude ranges from -180 to 180 degrees (or 0 to 360)
self.lon = np.linspace(-180, 180, N_lon, endpoint=False)
# Create 2D arrays of lat and lon values
self.lon_grid, self.lat_grid = np.meshgrid(self.lon, self.lat)
# Calculate grid spacing in meters
# Circumference at a given latitude is 2 * pi * radius * cos(latitude)
# Grid spacing in longitude direction changes with latitude
self.delta_lat = np.pi * radius / (N_lat - 1)
self.delta_lon = (2 * np.pi * radius / N_lon) * np.cos(np.deg2rad(self.lat_grid))
self.N_lat = N_lat
self.N_lon = N_lon
def get_coordinates(self):
""" Get the spherical coordinates of the grid points. """
# Convert degrees to radians for calculations
lat_rad = np.deg2rad(self.lat_grid)
lon_rad = np.deg2rad(self.lon_grid)
# Convert lat/lon to Cartesian coordinates for a sphere
x = self.radius * np.cos(lat_rad) * np.cos(lon_rad)
y = self.radius * np.cos(lat_rad) * np.sin(lon_rad)
z = self.radius * np.sin(lat_rad)
return x, y, z
def get_grid_spacing_meters(self):
""" Get the grid spacing in meters for both latitude and longitude. """
return self.delta_lat, self.delta_lon
# Example usage:
N_lat = 180 # Number of latitude grid points
N_lon = 360 # Number of longitude grid points
grid = Grid(N_lat, N_lon)
class grid:
""" Creates grid for ocean model
Args:
-------
N: Number of grid points (NxN)
L: Length of domain
"""
def __init__(self, N, L):
self.deltax = L / N
self.deltay = L / N
self.xs = np.arange(0-self.deltax/2, L+self.deltax/2, self.deltax)
self.ys = np.arange(-L-self.deltay/2, L+self.deltay/2, self.deltay)
self.N = N
self.L = L
self.Nx = len(self.xs)
self.Ny = len(self.ys)
self.grid, _= np.meshgrid(self.ys, self.xs, sparse=False, indexing='ij')
class windfield:
"""Calculates wind velocity
Args:
------
Grid: class grid()
option: zero, or single gyre
"""
def __init__(self, grid, option = "zero"):
if option == "zero":
self.u = np.zeros((grid.grid.shape))
self.v = np.zeros((grid.grid.shape))
if option == "single_gyre":
u, v = PointVortex(grid)
self.u = u
self.v = v
import matplotlib.pyplot as plt
import cartopy.crs as ccrs
import cartopy.feature as cfeature
from ipywidgets import interact, IntSlider
import numpy as np
def plot_temperature_distribution(T, grid):
# Create a figure with an axes set with a projection
fig = plt.figure(figsize=(12, 6))
ax = fig.add_subplot(1, 1, 1, projection=ccrs.PlateCarree())
ax.add_feature(cfeature.COASTLINE)
ax.add_feature(cfeature.BORDERS, linestyle=':')
# Set the extent (min longitude, max longitude, min latitude, max latitude)
# Plot the temperature data
mesh = ax.pcolormesh(grid.lon_grid, grid.lat_grid, T,
cmap='coolwarm', transform=ccrs.PlateCarree())
# Add a colorbar
cbar = plt.colorbar(mesh, orientation='vertical', pad=0.02, aspect=50)
cbar.set_label('Temperature (°C)')
# Add titles and labels
plt.title('Ocean Surface Temperature')
ax.set_xlabel('Longitude')
ax.set_ylabel('Latitude')
plt.show()
def update_model(steps):
for _ in range(steps):
model.timestep() # Call the timestep method of your model
plot_temperature_distribution(model.T[-1], model.grid) # Plot the latest temperature distribution
# Initialize your model here with the initial conditions
# Example:
model = OceanModel(initial_temperature, deltat, windfield, grid, kappa)
# Create an interactive slider for number of timesteps
interact(update_model, steps=IntSlider(min=1, max=100, step=1, value=1, description='Time Steps'))
<function __main__.update_model(steps)>
Initialize
Initialize temperature field with rectangular non-zero box.
%matplotlib inline
import matplotlib.pyplot as plt
import cartopy.crs as ccrs
import cartopy.feature as cfeature
import numpy as np
from ipywidgets import interactive, IntSlider
# Assume your OceanModel class definition is here
def plot_temperature_distribution(model):
fig = plt.figure(figsize=(10, 5))
ax = fig.add_subplot(1, 1, 1, projection=ccrs.PlateCarree())
# Retrieve the latest temperature data from the model
T = model.T[-1]
# Clear the previous plot to ensure we do not overlay the plots
ax.clear()
# Plot the temperature distribution
temperature_plot = ax.pcolormesh(model.grid.lon_grid, model.grid.lat_grid, T,
transform=ccrs.PlateCarree(),
cmap='coolwarm')
# Add coastlines and borders for context
ax.coastlines()
ax.add_feature(cfeature.BORDERS)
# Add a color bar
cbar = plt.colorbar(temperature_plot, orientation='horizontal', pad=0.05)
cbar.set_label('Temperature (°C)')
# Set map extent (could be global or a specific region)
ax.set_global()
# Add gridlines
ax.gridlines(draw_labels=True, dms=True, x_inline=False, y_inline=False)
# Show the plot
plt.show()
def timestep_and_plot(steps):
for _ in range(steps):
model.timestep() # Call the timestep method of your model
plot_temperature_distribution(model) # Plot the latest temperature distribution
# Initialize your model here with the initial conditions
# Example:
model = OceanModel(initial_temperature, deltat, windfield, grid, kappa)
# Create an interactive widget to control the number of timesteps
slider = IntSlider(min=1, max=100, step=1, value=1, description='Time Steps')
interactive_plot = interactive(timestep_and_plot, steps=slider)
display(interactive_plot)
my_grid = grid(N = 11, L = 6e6)
my_wind = windfield(my_grid, option = "zero")
my_temp = np.zeros((my_grid.grid.shape))
my_temp[9:13, 4:8] = 1
my_grid.grid.shape
(23, 12)
plt.pcolor(my_grid.xs, my_grid.ys, my_temp)
plt.colorbar(label = "Temperature in °C");
plt.ylabel("Kilometers Latitude");
plt.xlabel("Kilometers Longitude");
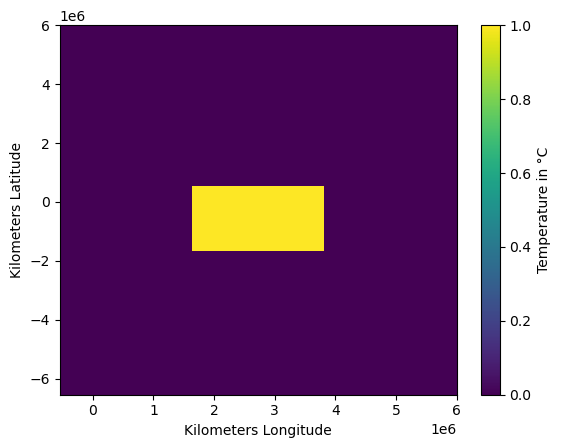
deltat = 12*60*60 # 12 Hours
def plot_func(steps, temp, deltat, windfield, grid, kappa, anomaly = False):
my_model = OceanModel(T=temp,
deltat=deltat,
windfield=windfield,
grid=grid,
kappa = kappa)
for step in range(steps):
my_model.timestep()
plt.figure(figsize=(8,8))
lon, lat = np.meshgrid(grid.xs, grid.ys)
if anomaly == True:
plt.pcolor(my_grid.xs, my_grid.ys, my_model.T[-1] - my_model.T[-2], cmap = plt.cm.RdBu_r,
vmin = -0.001, vmax = 0.001)
else:
plt.pcolor(my_grid.xs, my_grid.ys, my_model.T[-1], vmin = 0, vmax = 1)
plt.quiver(lon, lat, windfield.u, windfield.v)
plt.colorbar(label = "Temperature in °C");
plt.ylabel("Kilometers Latitude");
plt.xlabel("Kilometers Longitude");
plt.title("Time: {} days".format(int(step/2)))
plt.show()
interact(plot_func,
temp = fixed(my_temp),
deltat = fixed(deltat),
windfield = fixed(my_wind),
grid = fixed(my_grid),
kappa = fixed(1e4),
anomaly = widgets.Checkbox(description = "Anomaly", value=False),
steps = widgets.IntSlider(description="Timesteps", value = 2, min = 1, max = 10000, step = 1));
Create single gyre windfield#
def diagnose_velocities(stream, G):
dx = np.zeros((G.grid.shape))
dy = np.zeros((G.grid.shape))
dx = (stream[:, 1:] - stream[:, 0:-1])/G.deltax
dy = (stream[1:, :] - stream[0:-1, :])/G.deltay
u = np.zeros((G.grid.shape))
v = np.zeros((G.grid.shape))
u = 0.5*(dy[:, 1:] + dy[:, 0:-1])
v = -0.5*(dx[1:, :] + dx[0:-1, :])
return u, v
def impose_no_flux(u, v):
u[0, :] = 0; v[0, :] = 0;
u[-1,:] = 0; v[-1,:] = 0;
u[:, 0] = 0; v[:, 0] = 0;
u[:,-1] = 0; v[:,-1] = 0;
return u, v
def PointVortex(G, omega = 0.6, a = 0.2, x0=0.5, y0 = 0.):
x = np.arange(-G.deltax/G.L, 1 + G.deltax / G.L, G.deltax / G.L).reshape(1, -1)
y = np.arange(-1-G.deltay/G.L, 1+ G.deltay / G.L, G.deltay / G.L).reshape(-1, 1)
r = np.sqrt((y - y0)**2 + (x - x0)**2)
stream = -omega/4*r**2
stream[r > a] = -omega*a**2/4*(1. + 2*np.log(r[r > a]/a))
u, v = diagnose_velocities(stream, G)
u, v = impose_no_flux(u, v)
u = u * G.L; v = v * G.L
return u, v
my_wind = windfield(my_grid, option="single_gyre")
plt.quiver(my_wind.u, my_wind.v)
<matplotlib.quiver.Quiver at 0x134f329b0>
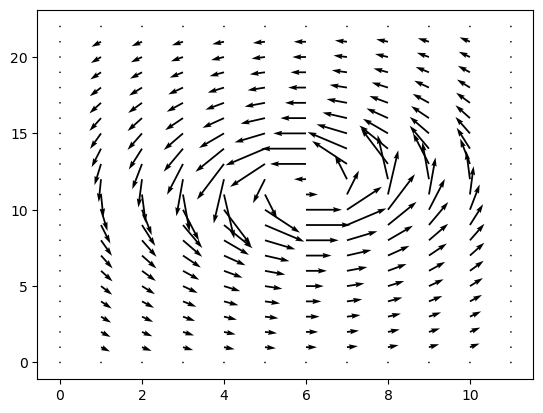
my_temp = np.zeros((my_grid.grid.shape))
my_temp[8:13, :] = 1
plt.pcolor(my_temp)
<matplotlib.collections.PolyCollection at 0x134f30190>
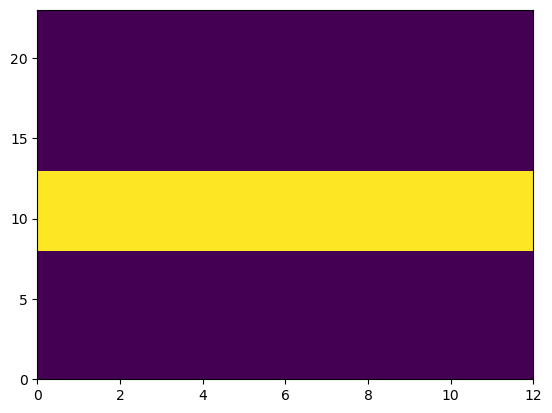
my_model = OceanModel(T=my_temp,
deltat=deltat,
windfield=my_wind,
grid=my_grid,
kappa = 1e4)
interact(plot_func,
temp = fixed(my_temp),
deltat = fixed(deltat),
windfield = fixed(my_wind),
grid = fixed(my_grid),
kappa = widgets.IntSlider(description="kappa", value = 1e4, min=1e3, max = 1e5, step = 100),
anomaly = widgets.Checkbox(description = "Anomaly", value=False),
steps = widgets.IntSlider(description="Timesteps", value = 1, min = 1, max = 1000, step = 1));
Optional: Create two gyres!